Dublin Airport
Optimizing Staff Rostering with Prediction Models
Dublin Airport (DUB) is state-owned and operated by DAA (formerly Dublin Airport Authority). In 2022, Dublin Airport served 28 million passengers, surpassing expectations for international travel compared to pre-pandemic levels (231% increase over 2021; 85% recovery of 2019 levels), and working with over 43 airline partners, Dublin Airport provides service to 180 destinations. The DAA data and analytics team, housed under the chief information officer, works directly with IT staff to operate and update business systems. DAA uses advanced analytics to forecast passenger volumes based on arrival patterns, flight schedules, and seasonal information to predict the number of passengers every day and provide recommendations for the number of security screening lanes to open and staff required. The data science/engineering team is successfully integrating the outputs from prediction models directly into the staff rostering system, which helps plan employee schedules and rosters to meet demand and operate the airport more efficiently.
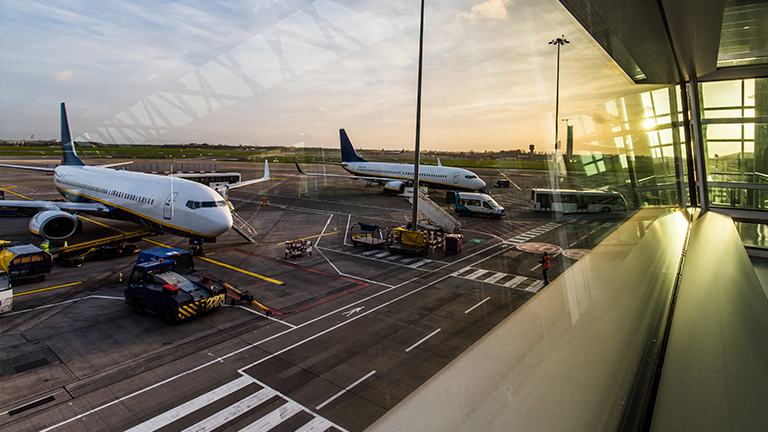
Airport Demographics
Western Europe
DAA
19,000 Employees
Data Analytics Capabilities at DAA
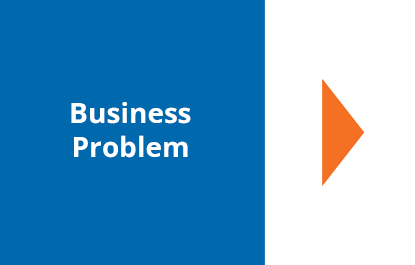
- Dublin Airport set out specific milestones for continuous improvements related to the passenger experience.
- The airport experienced passenger congestion that led to increased wait times at security screening checkpoints.
- Government imposed regulatory penalties for security screening that exceeded 30-minute wait times.
- Airport needed to determine the number of security lanes to open and staff required to meet passenger demand.
- Airport needed to plan staff rosters (schedules) to operate the airport efficiently according to the level of passenger demand.
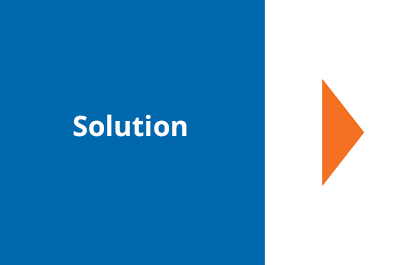
- DAA uses advanced analytics and statistical models of past and current data patterns to predict future patterns.
- Dublin Airport developed a comprehensive research passenger profiling program (passenger tracking).
- Forecasting models predict the number of passengers every day based on arrivals, flight schedules, and season.
- Machine learning (ML) algorithms are deployed to improve model accuracy and make improvements in security.
- Rostering system incorporates staff preferences matched with expected peaks in passenger demand.
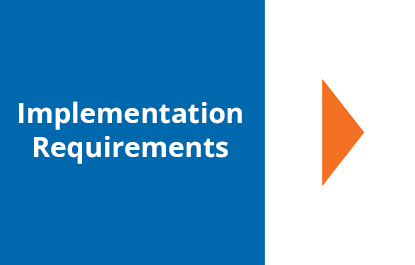
Technical Requirements
- The engineering team builds data platforms and corporate data warehouses to store and organize curated data.
- Data is passed to the analytics platform to calculate metrics that are viewable with drag-and-drop dashboards.
- Prediction models that forecast passenger demand on any given day by general trends and seasonality.
- Integrated process for using historical and current data to make real-time decisions (e.g., number of security lanes).
- Rostering system that integrates data from multiple sources, including staff preferences, shift duration, and demand.
Human Capital Requirements
- Network of strategic partners to manage demand and specific skillsets in data analytics/science/engineering.
- Product owners to provide domain expertise and perspective (e.g., security areas, parking, concessions).
- Individuals who are responsible and accountable for what happens as a result of the model forecasts (e.g., wait times).
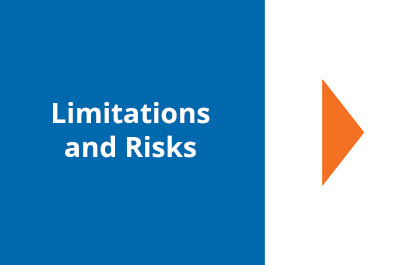
Data and Technology Limitations
- Predicting passenger demand is volatile as the number of passengers throughout the day can vary from day to day.
- Basic personnel numbers inform supply and need to be integrated to control supply throughput via staff rosters.
- With forecasting, not all errors are equal (e.g., root mean square error) and some models are more risk averse (e.g., more staff).
- Post-pandemic prediction models use historical demand data but weigh current/real-time information more heavily.
People and Process Limitations
- Irish government regulations impose fines for customer wait times for queues exceeding 30 minutes ($100,000).
- Level of risk tolerance and willingness to implement analytics solutions differed across the airport divisions.
- Explaining over- vs under-forecasting to stakeholders and selecting business-centric metrics to report out.
- The COVID-19 pandemic altered airport operations, and historical demand was not predictive for current demand.
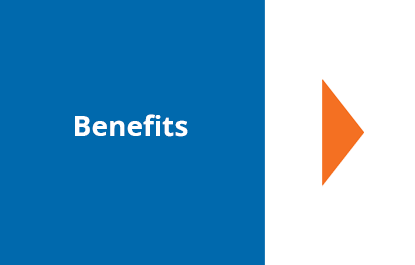
- The analytics team works directly with IT staff responsible for operating and upgrading key business systems.
- Forecasting passenger demand can reduce wait times and improve the quality of passenger experience.
- Passenger forecasts are essential for determining the number of security lanes to open and the staff required.
- Prediction models were integrated into the staff rostering system to meet the anticipated passenger demand.
- Optimizing the match between supply and demand increased operational efficiency and reduced wait times.
Change Management Strategies
- DAA made a strategic decision in 2014 to create a data culture and invest in new operating models, technical platforms, and analytics talent across the organization.
- Engaging with airport leadership on analytics early in the project helped to align the analytics objectives with business objectives.
- Division product owners identified the business problem, prioritized and signed off on business requirements, and aligned the analytics goals to business objectives.
- Growth in data generation requires investment in data capabilities to manage increased volume (e.g., big-data engineering and cloud-based solutions).
- Investments in modern data and analytics platforms, increased computing power to process data, and advanced tools to derive actionable insights (e.g., ML, artificial intelligence).
Greatest Challenges & Lessons Learned
Challenges
- User adoption is a key challenge to overcome for implementing analytics solutions.
- Passenger queueing (security) users are generally more risk averse and slower to adopt change.
- Creating model outputs that can be seamlessly ingested by business tools in the analytics workflow.
- Proliferation of sensor devices and getting an end-to-end view of operational efficiency at the airport is critical.
Lessons
- Leadership support is crucial to ensuring engagement with internal stakeholders, airport staff, and IT.
- Working collaboratively with business stakeholders is key to determining high-value business use-cases.
- Employees personally invested in forecasting had more confidence in the data models over time.
- Airports need to continually adapt to meet new data challenges by focusing on the right level of priorities.
Impact to Organization
Dublin Airport (DAA) is a data-driven organization with mature analytics capabilities. The DAA data and analytics team works with stakeholders in airport divisions to understand business challenges where analytics can increase operational efficiency and improve the passenger experience. Forecasting the passenger demand throughout the day provides updated forecasting models to optimize staff scheduling and reduce wait times at security areas. A key focus for the future is to have model outputs consumed by business tools seamlessly as part of the analytics workflow. At DAA, data analytics is viewed as a flexible, dynamic process, where experimentation, innovation, and creativity are encouraged.