Maturity Model and Evaluation Matrix
Framing the analytics maturity of an organization as a series of stages can provide guidance to airports at different levels about the next steps in adopting data analytics for their specific needs (Davenport 2018). As traditional businesses, airports can be slow to change and therefore may seek to expand their analytics capabilities incrementally over time. This process can take different pathways. The different stages of analytics maturity can be viewed by selecting each tab in Figure 1 below.
Figure 1. Stages of Analytics Maturity
Analytics Formation: At this stage, the airport has no enterprise perspective on data or analytics. Senior leadership has little awareness of or interest in analytics, and there is no targeting of analytics opportunities. The data resources are inconsistent and of relatively low quality. Analytical skills are low and attached to specific functions. Simple visual analytics are produced using standard desktop office applications (Excel). Basic reporting tools provide descriptive measures of central tendency and variability to identify data trends.
Localized Analytics: As an organization, the airport has islands of data, technology, and expertise, but much usable data is stored in functional silos. Senior leadership does not discuss data management, and individual analytics projects are directed at business areas that are typically low in strategic importance. Local analytics leaders emerge, but with little connection to analysts with a mixture of skills in statistical modeling, predictive analytics, programming languages, data science, or database management, which is required at higher levels of analytics maturity. Data is stored in spreadsheets or databases that can be queried. Statistical packages are used to obtain descriptive analytics with variability and confidence intervals, and to perform correlations and linear regression.
Analytical Aspirations: At the aspirational level, senior leaders recognize the importance of analytics capabilities and analytics initiatives are focused on a small set of important business processes. The enterprise has an analytics plan in place to develop the infrastructure, platform, and tools for analytics. Data is integrated in a data warehouse or data lake. Analysts provide key talent and are focused on important business areas. Simple predictive analytics models (e.g., regression, classification, clustering) are used for forecasting.
Analytical Organizations: In a data-driven organization, analytics are integral to strategic plans and analytical capabilities are being actively expanded. Data, technology, and analysts are managed from an enterprise perspective. Quality data are integrated and stored in cloud-based data warehouses or data lakes that may be managed by IT. Analytics initiatives are focused on key high value, high impact business areas, with some coordination across functional divisions or operational units. Highly skilled analysts are recruited and engaged to deploy advanced predictive analytics methods and discover insights from data using statistical and machine learning (ML) algorithms, model optimization, sentiment analysis, and text or image analytics.
Analytical Competitors: The culture of analytics is pervasive across the enterprise. Organizational leaders behave analytically and are engaged with the competitive advantage of analytics. Analytical resources are focused on enterprise priorities. Data is managed as a strategic asset, with new data and metrics being continuously identified. A sophisticated, enterprise-wide big data and analytics architecture is in place. Professional analysts are cultivated, and the analytics team is separate from IT. Prescriptive and autonomous analytics are implemented using automated ML and artificial intelligence (AI), ensemble models, and deep learning neural networks.
Figure 2. Stage Model of Analytics Maturity
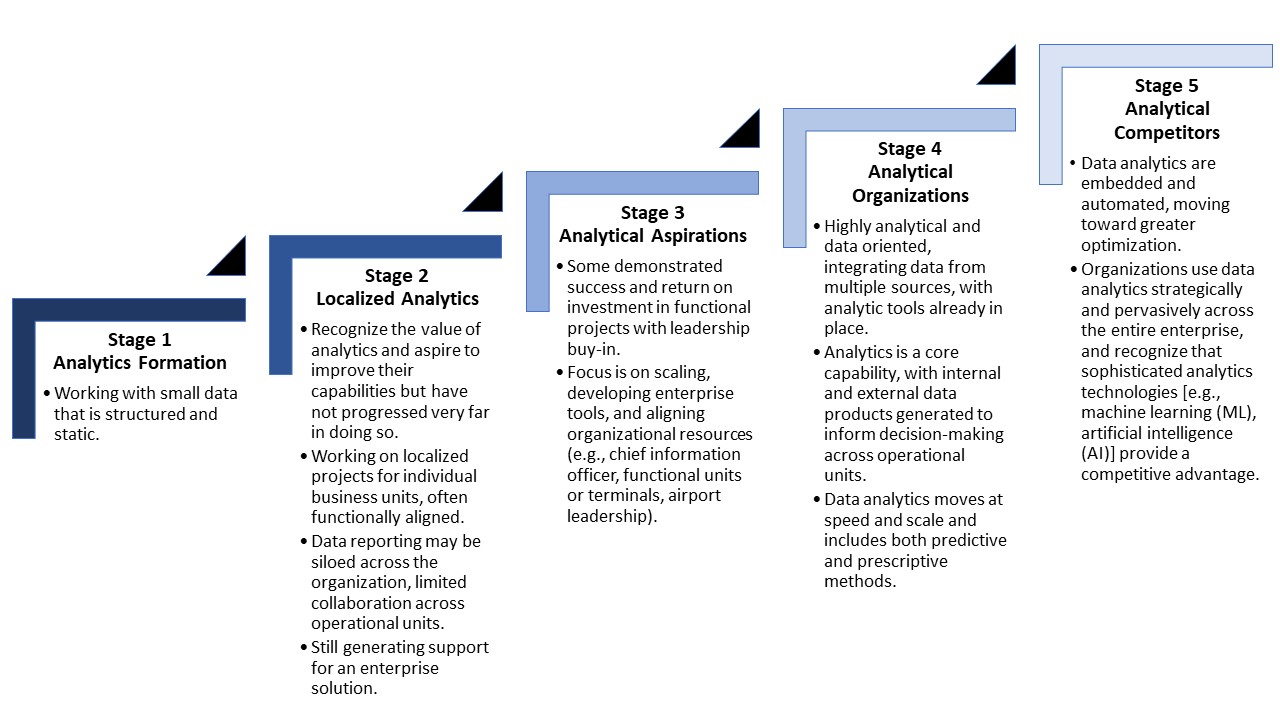
Estimating Your Organizational Analytics Maturity
The following set of questions provides examples of 10 key data management and analytics practices for estimating the approximate maturity of your organization. These questions provide an opportunity to reflect on different aspects of your analytics program. Your responses will be used to suggest activities for advancing your organization’s analytics maturity.
Steps that Airports Can Take to Progress in their Analytics Maturity
The maturity model describes data and analytics capabilities as distinct stages, but the progression of analytics development is often more incremental and continuous. In addition, an organization can be at a higher level of maturity in one area (e.g., analytics strategy and planning) and simultaneously at a lower level of maturity in another area (e.g., data management, analytics techniques). The section below describes several activities and practices that mark the transition between different levels of maturity. Airports can advance the development of their analytics maturity by incorporating best practices for data management, analytics procedures, and strategic planning in their analytics pathway.
Transition from Analytics Formation (Stage 1) to Localized Analytics (Stage 2)
Processes
- Gather important local data sources into functional data marts.
- Put important data into a data warehouse or data lake.
- Move toward statistical significance analyses and range-based forecasts.
Tools
- Partner with IT on common tool selection and data standards.
- Make visual analytics tools available; adopt predictive analytics tools.
- Explore optimization, web analytics, and other special-purpose tools.
Organization
- Encourage analytical leaders in functional airport divisions and business units.
- Manage data risks at the local level, across airport divisions.
- Encourage some airport divisions to adopt predictive models.
Transition from Localized Analytics (Stage 2) to Analytical Aspirations (Stage 3)
People
- Define analytical positions and use specialty recruiting.
- Build consensus around analytical targets and data needs.
- Ensure a central analytics team is well-trained in predictive and prescriptive analytics.
Processes
- Take systematic inventory of analytical opportunities by business area.
- Select applications for business problems with high analytical potential.
- Establish standards for data privacy and security.
Tools
- Identify a suite of analytics tools for use throughout the enterprise.
- Experiment with cloud-based analytics, data management, and big data applications.
- Experiment with statistical ML; explore text and sentiment analysis.
Organization
- Plan the groundwork for enterprise analytics strategy and priorities.
- Begin building enterprise analytical infrastructure incrementally.
- Motivate and reward cross-functional data contributions and management.
Transition from Analytical Aspirations (Stage 3) to Analytical Organizations (Stage 4)
Processes
- Work with high value, high impact business processes and their owners.
- Deploy a combined mix of analytic models using commercial and open-source tools.
- Conduct risk assessment for all analytical applications.
Tools
- Integrate internal and external resources in cloud-based data warehouses or data lakes.
- Implement self-service data platforms for extracting data and conducting analytics.
- Develop ML applications; explore deep learning and AI models.
Organization
- Develop and implement analytics IT roadmap for the entire enterprise.
- Engage senior leaders in building analytics capabilities: data, technology, human capital.
- Establish enterprise governance of technology and architecture for analytics.
Transition from Analytical Organizations (Stage 4) to Analytical Competitors (Stage 5)
Tools
- Move big data analytics to cloud-based platforms.
- Deploy automated ML and ensemble models using open-source tools.
- Explore the use of deep learning neural networks and AI toolsets for all types of data.
Organization
- Integrate analytics into the strategic planning process to shape business strategy.
- Manage analytical priorities, assets, and model review at the enterprise level.
- Extend analytical tools and infrastructure broadly and deeply across the enterprise.